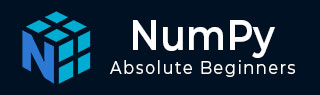
- NumPy Tutorial
- NumPy - Home
- NumPy - Introduction
- NumPy - Environment
- NumPy Arrays
- NumPy - Ndarray Object
- NumPy - Data Types
- NumPy Creating and Manipulating Arrays
- NumPy - Array Creation Routines
- NumPy - Array Manipulation
- NumPy - Array from Existing Data
- NumPy - Array From Numerical Ranges
- NumPy - Iterating Over Array
- NumPy - Reshaping Arrays
- NumPy - Concatenating Arrays
- NumPy - Stacking Arrays
- NumPy - Splitting Arrays
- NumPy - Flattening Arrays
- NumPy - Transposing Arrays
- NumPy Indexing & Slicing
- NumPy - Indexing & Slicing
- NumPy - Indexing
- NumPy - Slicing
- NumPy - Advanced Indexing
- NumPy - Fancy Indexing
- NumPy - Field Access
- NumPy - Slicing with Boolean Arrays
- NumPy Array Attributes & Operations
- NumPy - Array Attributes
- NumPy - Array Shape
- NumPy - Array Size
- NumPy - Array Strides
- NumPy - Array Itemsize
- NumPy - Broadcasting
- NumPy - Arithmetic Operations
- NumPy - Array Addition
- NumPy - Array Subtraction
- NumPy - Array Multiplication
- NumPy - Array Division
- NumPy Advanced Array Operations
- NumPy - Swapping Axes of Arrays
- NumPy - Byte Swapping
- NumPy - Copies & Views
- NumPy - Element-wise Array Comparisons
- NumPy - Filtering Arrays
- NumPy - Joining Arrays
- NumPy - Sort, Search & Counting Functions
- NumPy - Searching Arrays
- NumPy - Union of Arrays
- NumPy - Finding Unique Rows
- NumPy - Creating Datetime Arrays
- NumPy - Binary Operators
- NumPy - String Functions
- NumPy - Matrix Library
- NumPy - Linear Algebra
- NumPy - Matplotlib
- NumPy - Histogram Using Matplotlib
- NumPy Sorting and Advanced Manipulation
- NumPy - Sorting Arrays
- NumPy - Sorting along an axis
- NumPy - Sorting with Fancy Indexing
- NumPy - Structured Arrays
- NumPy - Creating Structured Arrays
- NumPy - Manipulating Structured Arrays
- NumPy - Record Arrays
- Numpy - Loading Arrays
- Numpy - Saving Arrays
- NumPy - Append Values to an Array
- NumPy - Swap Columns of Array
- NumPy - Insert Axes to an Array
- NumPy Handling Missing Data
- NumPy - Handling Missing Data
- NumPy - Identifying Missing Values
- NumPy - Removing Missing Data
- NumPy - Imputing Missing Data
- NumPy Performance Optimization
- NumPy - Performance Optimization with Arrays
- NumPy - Vectorization with Arrays
- NumPy - Memory Layout of Arrays
- Numpy Linear Algebra
- NumPy - Linear Algebra
- NumPy - Matrix Library
- NumPy - Matrix Addition
- NumPy - Matrix Subtraction
- NumPy - Matrix Multiplication
- NumPy - Element-wise Matrix Operations
- NumPy - Dot Product
- NumPy - Matrix Inversion
- NumPy - Determinant Calculation
- NumPy - Eigenvalues
- NumPy - Eigenvectors
- NumPy - Singular Value Decomposition
- NumPy - Solving Linear Equations
- NumPy - Matrix Norms
- NumPy Element-wise Matrix Operations
- NumPy - Sum
- NumPy - Mean
- NumPy - Median
- NumPy - Min
- NumPy - Max
- NumPy Set Operations
- NumPy - Unique Elements
- NumPy - Intersection
- NumPy - Union
- NumPy - Difference
- NumPy Random Number Generation
- NumPy - Random Generator
- NumPy - Permutations & Shuffling
- NumPy - Uniform distribution
- NumPy - Normal distribution
- NumPy - Binomial distribution
- NumPy - Poisson distribution
- NumPy - Exponential distribution
- NumPy - Rayleigh Distribution
- NumPy - Logistic Distribution
- NumPy - Pareto Distribution
- NumPy - Visualize Distributions With Sea born
- NumPy - Matplotlib
- NumPy - Multinomial Distribution
- NumPy - Chi Square Distribution
- NumPy - Zipf Distribution
- NumPy File Input & Output
- NumPy - I/O with NumPy
- NumPy - Reading Data from Files
- NumPy - Writing Data to Files
- NumPy - File Formats Supported
- NumPy Mathematical Functions
- NumPy - Mathematical Functions
- NumPy - Trigonometric functions
- NumPy - Exponential Functions
- NumPy - Logarithmic Functions
- NumPy - Hyperbolic functions
- NumPy - Rounding functions
- NumPy Fourier Transforms
- NumPy - Discrete Fourier Transform (DFT)
- NumPy - Fast Fourier Transform (FFT)
- NumPy - Inverse Fourier Transform
- NumPy - Fourier Series and Transforms
- NumPy - Signal Processing Applications
- NumPy - Convolution
- NumPy Polynomials
- NumPy - Polynomial Representation
- NumPy - Polynomial Operations
- NumPy - Finding Roots of Polynomials
- NumPy - Evaluating Polynomials
- NumPy Statistics
- NumPy - Statistical Functions
- NumPy - Descriptive Statistics
- NumPy Datetime
- NumPy - Basics of Date and Time
- NumPy - Representing Date & Time
- NumPy - Date & Time Arithmetic
- NumPy - Indexing with Datetime
- NumPy - Time Zone Handling
- NumPy - Time Series Analysis
- NumPy - Working with Time Deltas
- NumPy - Handling Leap Seconds
- NumPy - Vectorized Operations with Datetimes
- NumPy ufunc
- NumPy - ufunc Introduction
- NumPy - Creating Universal Functions (ufunc)
- NumPy - Arithmetic Universal Function (ufunc)
- NumPy - Rounding Decimal ufunc
- NumPy - Logarithmic Universal Function (ufunc)
- NumPy - Summation Universal Function (ufunc)
- NumPy - Product Universal Function (ufunc)
- NumPy - Difference Universal Function (ufunc)
- NumPy - Finding LCM with ufunc
- NumPy - ufunc Finding GCD
- NumPy - ufunc Trigonometric
- NumPy - Hyperbolic ufunc
- NumPy - Set Operations ufunc
- NumPy Useful Resources
- NumPy Compiler
- NumPy - Quick Guide
- NumPy - Cheatsheet
- NumPy - Useful Resources
- NumPy - Discussion
NumPy - Finding Unique Rows
Finding Unique Rows in NumPy Array
In NumPy, arrays can contain multiple rows of data, and sometimes you might want to identify rows that are unique, meaning they appear only once in the array. Finding unique rows involves determining which rows are distinct from others based on their content.
In NumPy, we can achieve this using the unique() function.
Using union1d() Function
The np.unique() function is commonly used to find unique elements in an array. When applied with the axis parameter, it can be used to find unique rows. Following is the syntax −
numpy.unique(a, axis=None, return_index=False, return_inverse=False, return_counts=False)
Where,
- a − It is the input array.
- axis − It is the axis along which to find unique values. Set to 0 for rows.
- return_index − It determines whether to return the indices of the first occurrences.
- return_inverse − It determines whether to return the indices that can reconstruct the array.
- return_counts − It determines whether to return the counts of unique values.
Example: Finding Unique Elements in a 1D Array
The simplest use of np.unique() function is to find unique elements in a one-dimensional array −
import numpy as np # Define a 1D array with duplicate values array = np.array([1, 2, 2, 3, 4, 4, 5]) # Find unique elements unique_elements = np.unique(array) print("Unique Elements:\n", unique_elements)
Following is the output obtained −
Unique Elements: [1 2 3 4 5]
Example: Unique Rows in a 2D Array
In the following example, we are using the unique() function to retrieve the unique rows in a 2D array, removing any duplicate rows −
import numpy as np # Define an array with duplicate rows array = np.array([ [1, 2, 3], [4, 5, 6], [1, 2, 3], [7, 8, 9] ]) # Find unique rows unique_rows = np.unique(array, axis=0) print("Unique Rows:\n", unique_rows)
This will produce the following result −
Unique Rows: [[1 2 3] [4 5 6] [7 8 9]]
Finding Unique Rows with Indexes
We can find the indices of the unique rows in the original array in NumPy by setting the return_index parameter to True in the unique() function.
Example
In this example, we are finding unique rows and their indices using the unique() function −
import numpy as np # Define an array with duplicate rows array = np.array([ [1, 2, 3], [4, 5, 6], [1, 2, 3], [7, 8, 9] ]) # Find unique rows and their indices unique_rows, indices = np.unique(array, axis=0, return_index=True) print("Unique Rows:\n", unique_rows) print("Indices of Unique Rows:\n", indices)
Following is the output of the above code −
Unique Rows: [[1 2 3] [4 5 6] [7 8 9]] Indices of Unique Rows: [0 1 3]
Reconstructing the Original Array
If you need to reconstruct the original array from the unique rows, you can use the indices returned by np.unique() function with the return_inverse parameter set to True. The inverse indices can be used to map back to the original data from the unique values.
Example
In this example, we are identifying unique rows in a NumPy array and their original indices using the unique() function. We then reconstruct the array using these indices to verify that the unique rows match the original array without duplicates −
import numpy as np # Define an array with duplicate rows array = np.array([ [1, 2, 3], [4, 5, 6], [1, 2, 3], [7, 8, 9] ]) # Find unique rows and their indices unique_rows, indices = np.unique(array, axis=0, return_index=True) # Reconstruct the original array using the indices reconstructed_array = array[np.sort(indices)] print("Reconstructed Array:\n", reconstructed_array)
The output obtained is as shown below −
Reconstructed Array: [[1 2 3] [4 5 6] [7 8 9]]
Counting Unique Rows
In addition to finding unique rows, you might want to count how many times each unique row appears in the array. In NumPy, you can achieve this by setting the return_counts parameter to True in the unique() function.
This is particularly useful when working with multi-dimensional arrays where each row represents a record or observation.
Example
In the following example, we retrieve the count of each unique row in the original array using the unique() function −
import numpy as np # Define an array with duplicate rows array = np.array([ [1, 2, 3], [4, 5, 6], [1, 2, 3], [7, 8, 9] ]) # Find unique rows and their counts unique_rows, counts = np.unique(array, axis=0, return_counts=True) print("Unique Rows:\n", unique_rows) print("Counts of Each Row:\n", counts)
After executing the above code, we get the following output −
Unique Rows: [[1 2 3] [4 5 6] [7 8 9]] Counts of Each Row: [2 1 1]
Multi-dimensional Arrays
For multi-dimensional arrays, you can use np.unique() function to find unique rows by setting the axis parameter to 0. To handle unique values across all dimensions, you can use the default settings.
Example
In the example below, we flatten the 3D array into 2D and then find unique rows using the unique() function −
import numpy as np # Define a 3D array array = np.array([ [[1, 2], [3, 4]], [[1, 2], [5, 6]], [[1, 2], [3, 4]] ]) # Flatten the 3D array to 2D for uniqueness check array_2d = array.reshape(-1, array.shape[-1]) # Find unique rows in the flattened array unique_rows = np.unique(array_2d, axis=0) print("Unique Rows in 3D Array:\n", unique_rows)
The result produced is as follows −
Unique Rows in 3D Array: [[1 2] [3 4] [5 6]]