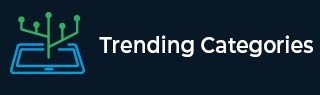
Data Structure
Networking
RDBMS
Operating System
Java
MS Excel
iOS
HTML
CSS
Android
Python
C Programming
C++
C#
MongoDB
MySQL
Javascript
PHP
Physics
Chemistry
Biology
Mathematics
English
Economics
Psychology
Social Studies
Fashion Studies
Legal Studies
- Selected Reading
- UPSC IAS Exams Notes
- Developer's Best Practices
- Questions and Answers
- Effective Resume Writing
- HR Interview Questions
- Computer Glossary
- Who is Who
What are the challenges of Outlier detection?
An outlier is a data object that deviates essentially from the rest of the objects, as if it were produced by a different structure. For ease of presentation, it can define data objects that are not outliers as “normal” or expected information. Similarly, it can define outliers as “abnormal” data.
Outliers are data components that cannot be combined in a given class or cluster. These are the data objects which have several behaviour from the general behaviour of different data objects. The analysis of this kind of data can be important to mine the knowledge.
There are various challenges of outlier detection is as follows −
Modeling normal objects and outliers effectively − Outlier detection element largely based on the modeling of normal (nonoutlier) objects and outliers. This is slightly because it is complex to enumerate some available normal behaviors in an application.
The border among data normality and abnormality (outliers) is not clear cut. Instead, there can be a broad range of gray application. Consequently, while various outlier detection techniques assign to each object in the input information set a label of either “normal” or “outlier,” other approach assign to each object a score calculating the “outlier-ness” of the object.
Application-specific outlier detection − It is selecting the similarity/distance measure and the relationship model to define data objects is essential in outlier detection. Unfortunately, such choices are software-dependent. There are several applications can have multiple requirements.
Handling noise in outlier detection − Outliers are different from noise. It is known that the quality of real information sets influence to be poor. Noise provide unavoidably exists in data collected in several applications. Noise can be show as deviations in attribute values or make smooth as missing values.
Low data quality and the existence of noise carry a huge challenge to outlier detection. They can deceive the information, blurring the differentiation among normal objects and outliers. Furthermore, noise and missing information can “hide” outliers and decrease the effectiveness of outlier detection an outlier can occur “disguised” as a noise point, and an outlier detection approach can erroneously recognize a noise point as an outlier.
Understandability − In some application methods, a user can required to not only detect outliers, but also learn why the detected objects are outliers. It can combine the understandability requirement, an outlier detection techniques has to support some reasons of the detection.
For instance, a statistical approach can be used to validate the degree to which an object can be an outlier depends on the likelihood that the object was created by the same structure that generated the majority of the records. The smaller the likelihood, the more unlikely the object was produced by the same structure, and the more acceptable the object is an outlier.