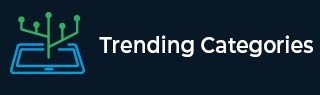
Data Structure
Networking
RDBMS
Operating System
Java
MS Excel
iOS
HTML
CSS
Android
Python
C Programming
C++
C#
MongoDB
MySQL
Javascript
PHP
Physics
Chemistry
Biology
Mathematics
English
Economics
Psychology
Social Studies
Fashion Studies
Legal Studies
- Selected Reading
- UPSC IAS Exams Notes
- Developer's Best Practices
- Questions and Answers
- Effective Resume Writing
- HR Interview Questions
- Computer Glossary
- Who is Who
Karl Pearson Coefficient Of Correlation
Coefficient of Correlation
Coefficients of correlation are generally used in statistics to measure a relationship between two variables. The correlation generally shows a specific value of the degree of a linear relationship between two variables, say X and Y. There are many types of correlation coefficients that are used in statistics. However, Karl Pearson’s correlation (also known as Pearson’s R) is the correlation coefficient that is most frequently used in linear regression.
Types of Correlation Coefficient
Depending on the direction of the relationship between variables, correlation can be of three types, namely –
Positive Correlation (0 to +1)
Negative Correlation (0 to -1)
Zero Correlation (0)
Positive Correlation (0 to +1)
In this case, the direction of change or the direction of occurrence of two functions between X and Y is the same. For instance, an increase in the application of coal leads to an increase in the number of power one burns in a coal-powered rail engine.
Negative Correlation (0 to -1)
In this case, the direction of change between X and Y variables is opposite. For example, with the increase in the price of commodities, its demand goes down.
Zero Correlation (0)
In the case of zero correlation, there is no relationship between the variables For instance, an increase in intake of foods has no impact on one’s driving ability.
Pearson’s Coefficient Correlation
Karl Pearson’s coefficient of correlation is an exclusively used mathematical method in which the numerical representation is used to measure the level of relation between two linearly related variables. The coefficient of correlation is expressed by “r”.
Actual Mean Method
In the actual mean method, it is expressed as:
$\mathrm{r\:=\:\frac{\sum\:(X\:-\:\bar{X})\:(Y\:-\:\bar{Y})}{\sqrt{\sum\:(X\:-\:\bar{X})^{2}}\:\sqrt{\sum\:(Y\:-\:\bar{Y})^{2}}}}$
Where, $\mathrm{\bar{X}\:=\:mean\:of\:X\:variable}$
$\mathrm{\bar{Y}\:=\:mean\:of\:Y\:variable}$
This method of expression of Pearson's correlation is known as the actual mean method.
Assumed Mean Method
There is another method known as an assumed mean method to express the correlation coefficient. The assumed mean method is expressed as:
Assumed Mean Method:
$\mathrm{d_{x}\:=\:X\:-\:A}$
$\mathrm{d_{y}\:=\:Y\:-\:A}$
$\mathrm{r\:=\:\frac{N\:\sum\:d_{x}\:d_{y}\:-\:(\sum\:d_{x})\:(\sum\:d_{y})}{\sqrt{N\:\sum\:d_x^2\:-\:(\sum\:d_{x})^{2}\:-\:\sqrt{N\:\sum\:d_y^2\:-\:(\sum\:d_{y})^{2}}}}}$
In this Karl Pearson Correlation formula,
dx = x-series’ deviation from assumed mean, wherein (X - A)
dy = Y-series’ deviation from assumed mean = ( Y - A)
Σdx. dy implies the summation of multiple dx and dy.
Σdx2 is the summation of the square of dx.
Σdy to the power 2 is the summation of the square of dy.
Σdx is the summation of the X-series' deviation.
Σdy is a summation of the Y-series, and
N is the number of observations in pairs.
Step Deviation Method
Is expressed as
$\mathrm{r\:=\:\frac{dX^{'}\:dY^{'}\:-\:\frac{\sum\:d^{'}\:X\:\sum\:d\:Y^{'}}{N}}{\sqrt{(\sum\:d\:x^{1})^{2}}\:-\:\frac{(\sum\:d\:x^{1})^{2}}{N}\:.\:(\sum\:d\:y^{'})^{2}\:\frac{(\sum\:d\:y^{'})^{2}}{N}}}$
In this particular Karl Pearson Method,
dx′=dxC1dx′=dxC1
dy′=dyC2dy′=dyC2
C1 = Common factor for series -x
C2 = Common factor for series -y
dx is the x-series’ deviation from the assumed mean, where (X - A)
dy is Y-series’ deviation from the assumed mean, where ( Y - A)
Σdx. dy implies the summation of multiple dx and dy.
Σdx2 is the summation of the square of dx.
Σdy2 is the summation of the square of dy.
Σdx is the summation of the X-series' deviation.
Σdy is the summation of the Y-series.
N is the number of observations in pairs.
The main features of the Karl Pearson Coefficient of correlation
There is no unit of Correlation Coefficient (r).
If r has a positive value, it signifies that both X and Y are moving in the same direction.
If r has a negative value, it indicates that X and Y are moving in opposite directions.
X and Y are said to be not correlated if the value of r is 0.
r with a high value indicates a strong linear relationship between two variables.
r with a low value shows a weak relationship between two variables.
Correlation between two variables is termed as perfect if the value of r is either +1 or -1.
Assumptions of Karl Pearson Coefficient Correlation
While calculating the Karl Pearson Correlation, a few assumptions must be made.
The following are the two main assumptions:
There is always a linear relationship between any two variables.
The outliers must be kept to a minimum range or removed totally.
Outliers are extra unusable data that contrast drastically with the rest of the data. It might signify the extreme data which actually do not fit in the set. One can spot an outlier by plotting the data on graph paper and looking for any extreme study. Outliers do not fall on Pearson’s graph and are found at extreme points of the graph.
Examples of Pearson’s Coefficient
When a correlation coefficient is (1), that means the correlation is positive. That is, for every increase in one variable, there is a positive increase in the other in a fixed proportion. For example, changes in shoe sizes according to the length of the feet are an example of perfect (almost) correlations.
The correlation coefficient is (-1) means for every positive increase in one variable, there is a proportional negative decrease in the other variable. For example, the reduction in the quantity of gas in a gas tank shows a perfect (almost) inverse correlation with speed.
When a correlation coefficient is (0), the two variables are not related.
When to use the Pearson correlation coefficient
The Pearson correlation coefficient (r) is a good choice when all of the following are true:
Both variables are quantitative: A different method must be chosen if any of the variables are qualitative.
The variables are normally distributed: A histogram of each variable can be prepared to verify whether the distributions of the variables are approximately normal. If the variables are a little non-normal, there is no problem..
The data have no outliers: Outliers are observations that do not follow the same patterns as the remaining data. A scatterplot is a good way to check for outliers—it is important to look for points that are extremely distant from the others.
The relationship is linear: “Linear” indicates that the relationship between the two variables is presentable more or less by a straight line. Checking whether the relationship is linear can be done by software nowadays.
Conclusion
The Karl Pearson coefficient of Correlation is a major tool in statistics to study the regression of linear variables. It helps users in many ways. As the study is dependent on linear variables, it is easy to use the results of the study. The use of this coefficient is widespread and it is also used in day-to-day lives. In order to get a better idea in many spaces such as shoe-making to gas filling, the coefficient can play a major role. Therefore, the study of Karl Pearson's coefficient of correlation should be made by one and all who want to check statistical models of bivariate studies.
FAQs
Q1. What is meant by coefficients of correlation?
Ans. Coefficients of correlation are generally used in statistics to measure a relationship between two variables. The correlation generally shows a specific value of the degree of a linear relationship between two variables, say X and Y.
Q2. What are the assumptions in the calculation of Karl Pearson's coefficient of correlation?
Ans. While calculating the Karl Pearson Correlation, a few assumptions must be made.
The following are the two main assumptions:
There is always a linear relationship between any two variables.
The outliers must be kept to a minimum range or removed totally.