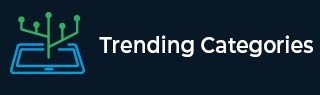
Data Structure
Networking
RDBMS
Operating System
Java
MS Excel
iOS
HTML
CSS
Android
Python
C Programming
C++
C#
MongoDB
MySQL
Javascript
PHP
Physics
Chemistry
Biology
Mathematics
English
Economics
Psychology
Social Studies
Fashion Studies
Legal Studies
- Selected Reading
- UPSC IAS Exams Notes
- Developer's Best Practices
- Questions and Answers
- Effective Resume Writing
- HR Interview Questions
- Computer Glossary
- Who is Who
How can Tensorflow and Estimator be used with Boosted trees to train and evaluate the model?
Tensorflow and Estimator can be used with Boosted trees to train and evaluate the model with the help of ‘BoostedTreesClassifier’. This estimator is used with the ‘train’ method and the ‘evaluate’ method to train and evaluate the dataset respectively.
Read More: What is TensorFlow and how Keras work with TensorFlow to create Neural Networks?
We will use the Keras Sequential API, which is helpful in building a sequential model that is used to work with a plain stack of layers, where every layer has exactly one input tensor and one output tensor.
A neural network that contains at least one layer is known as a convolutional layer. We can use the Convolutional Neural Network to build learning model.
We are using the Google Colaboratory to run the below code. Google Colab or Colaboratory helps run Python code over the browser and requires zero configuration and free access to GPUs (Graphical Processing Units). Colaboratory has been built on top of Jupyter Notebook.
We will see how a gradient boosting model can be trained using decision trees and tf.estimator API.
Let us understand how Estimators can be used. An Estimator is TensorFlow's high-level representation of a complete model. It is designed for easy scaling and asynchronous training.
We will train a logistic regression model using the tf.estimator API. The model is used as a baseline for other algorithms. We use the titanic dataset with the goal of predicting passenger survival, given characteristics such as gender, age, class, etc.
Estimators use feature columns to describe how the model would interpret the raw input features. An Estimator expects a vector of numeric inputs, and feature columns will help describe how the model should convert every feature in the dataset. Selecting and using the right set of feature columns is essential to learning an effective model. A feature column can be one of the raw inputs in the original features dict, or a new column created using transformations that are defined on one or multiple base columns.
Boosted Trees models are considered the most popular and effective machine learning approaches for regression as well as classification. It is an ensemble technique which combines the predictions from many (10s or 100s or 1000s) tree models.
The BoostedTreesRegressor and classification, supports BoostedTreesClassifier. The goal is to predict a class, that is survive or not survive.
Example
print("Using boosted trees") print("Data fits into memory, hence entire dataset per layer is used as a single batch") print("This would be quick") n_batches = 1 est = tf.estimator.BoostedTreesClassifier(feature_columns, n_batches_per_layer=n_batches) print("The model stops training once the number of trees specified is built") est.train(train_input_fn, max_steps=100) print("The model is evaluated") result = est.evaluate(eval_input_fn) clear_output() print(pd.Series(result))
Output
accuracy 0.833333 accuracy_baseline 0.625000 auc 0.875237 auc_precision_recall 0.859974 average_loss 0.405130 label/mean 0.375000 loss 0.405130 precision 0.795699 prediction/mean 0.383989 recall 0.747475 global_step 100.000000 dtype: float64
Explanation
- Boosted Trees model is trained.
- The goal is to predict a class, which could be survive or not survive.
- For this purpose, BoostedTreesClassifier is used.