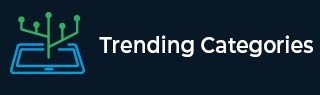
Data Structure
Networking
RDBMS
Operating System
Java
MS Excel
iOS
HTML
CSS
Android
Python
C Programming
C++
C#
MongoDB
MySQL
Javascript
PHP
Physics
Chemistry
Biology
Mathematics
English
Economics
Psychology
Social Studies
Fashion Studies
Legal Studies
- Selected Reading
- UPSC IAS Exams Notes
- Developer's Best Practices
- Questions and Answers
- Effective Resume Writing
- HR Interview Questions
- Computer Glossary
- Who is Who
Difference Between Cross-Sectional Analysis and Time Series Analysis
Although people consider cross-sectional and time-series analysis to be the same; however, they are totally different in many aspects.
Based on Use and Nature of Data
The main difference between cross-sectional and time series analysis is in their use of data. Moreover, the data collected for time series and cross-sectional analysis are different in nature too.
The difference between cross-sectional data and time-series data is that time-series data considers the same variables over a certain period of time, whereas cross-sectional data uses different data for a given point in time. It means that time-series data are stable, whereas the data used in the cross-sectional analysis are scattered.
Based on Observation Type
Time series data are an observation of data that are collected at specific intervals of time. Therefore, time-series data may be categorized into hourly, daily, monthly, quarterly, half yearly, and yearly. The idea is to check the similarity and differences of data recorded at different time periods.
Example − Suppose time series data is the daily opening prices of a share over a half-yearly period. It is notable that a too long or a too-short gap of observation of time series data may lead to erratic results. Therefore, the observations must be recorded in ideal gaps to avoid errors.
Cross-sectional data are observations of multiple subjects at one point in time.
Example − The value of opening prices of 10 selected stocks on a given date is an example of cross-sectional data. It is to be noted that cross-sectional data should have the same or similar characteristics for comparison. Items with different characteristics having different properties cannot be included in observation in the case of cross-sectional analysis.
Important Note − Time-series analysis is an analysis to determine the future pattern by considering the past and present conditions and then extending the trend to future conditions. Therefore, in the case of time-series data, the longer the interval between two data collection times, the better it is for the future prediction of the outcome.
Based on Future Data Forecast
Example 1 − Let’s say students who register for a given subject in a particular year. Note that there are specific data (the number of students and their presence cannot be altered) in the case of this time-series analysis. These data can be used to forecast future data patterns through time series analysis.
In the case of cross-sectional analysis, the most common example should consist of companies from the same industries so that errors in comparison can be avoided.
Example 2 − If we check the expense of R&D, the technology companies invest a huge amount in R&D in comparison to retail firms that do not have R&D centers.
Therefore, comparing R&D investments of technology and retail firms would lead to erratic results. However, selecting companies from retails alone can offer a good insight into the potential of the companies in R&D expenses. This is also applicable to technology firms analyzed alone.
Important Note − It is often erratic to take up subjects from varying domains to find out the cross-sectional results. As companies from different industries have different attributes, taking companies from different industries should be avoided for a more correct result of the cross-sectional analysis.
Conclusion
Both time series and cross-sectional data analysis are of importance to finance professionals. However, their aims and objectives are different. Whereas time series analysis is more apt to forecast a future condition, the cross-sectional analysis is better to find out the near-term future of the potential of a firm. Therefore, they should be used in accordance with the needs of the individuals and firms from time to time.